An integrated passive acoustic monitoring and deep learning pipeline for black-and-white ruffed lemurs (Varecia variegata) in Ranomafana National Park, Madagascar
Rainforest Connection & Arbimon’s Science Outreach Lead, Carly Batist, is the lead author of a new study in the American Journal of Primatology that used passive acoustic monitoring (PAM) and machine learning (ML) to study black-and-white ruffed lemurs in southeastern Madagascar.
Black-and-white ruffed lemurs are keystone species in Malagasy rainforests, as they fill vital pollinator and seed disperser roles. However, they are Critically Endangered and limited to fragments of the once-continuous eastern Malagasy rainforests, which makes them challenging to study. PAM is a promising method to overcome this and effectively provide data on species presence, distribution, and behavior, which can inform conservation efforts.
The study aimed to compare PAM to traditional survey methods, create a machine learning model to detect lemur calls from audio data (& compare to manual analysis), and explore lemur vocal patterns.
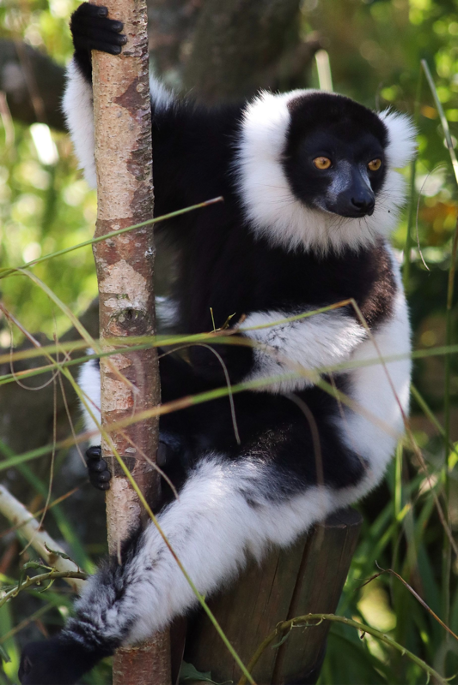
Data was collected in Ranomafana National Park, using passive acoustic recorders and simultaneous in-person behavioral observations. The authors then developed an automated ML call detection model and compared the results to those from manual detections.
The study concluded that the PAM+ML pipeline was more effective than the in-person observation+manual analysis pipeline with respect to time, cost, labor, and the benefit of generating a permanent dataset that can be re-analyzed. While PAM required a high upfront cost (to purchase recorders, batteries, etc.), this paid for itself after only 2 months in this study.

The study also provided the first quantitative, published evidence of nocturnal calling for ruffed lemurs! Interestingly, there was a particular peak in vocal activity (nocturnal & diurnal) during the short mating period.
While this study focused on ruffed lemurs in Madagascar, this workflow can be adapted for other primate species and range countries as well. The training data and model code has been open-sourced, serving as a resource for those interested in using ML methods. This study highlights the impressive potential of passive acoustic monitoring and machine learning as cost-efficient and scalable tools for biodiversity monitoring.